
The Business Process Modeling Notation (BPMN) has been established as a modeling standard in Business Process (BP) Management. Multiple demo examples are discussed to provide insights and making this connection based on commercial and non-commercial DES packages. This study aims to provide a step-wise tutorial for helping simulation users to create intelligent DES models by integrating them with Python. This integration makes the simulation modeling more intelligent and extends its applicability to a broader range of problems. Therefore, coupling these DES with external programming languages like Python offers additional mathematical operations and algorithmic flexibility. These tools are capable of modeling real-life systems with high accuracy, they generally fail to conduct advanced analytical analysis or complicated optimization (i.e.
#Anylogic pickup offset software#
Therefore, there are a variety of commercial (Simio, AnlyLogic, Simul8, Arena, etc.) and non-commercial software packages that enable users to take advantage of DES modeling. Discrete-even simulation (DES) is a common simulation approach to model time-dependent and complex systems. Simulation is an excellent tool to study real-life systems with uncertainty. This tutorial describes these methods, focusing on BO of composite objective functions, where one can observe and selectively evaluate individual constituents that feed into the overall objective and multi-fidelity BO, where one can observe cheaper approximations of the objective function by varying parameters of the evaluation oracle. We call these "grey-box" BO methods because they treat objective computation as partially observable and even modifiable, blending the black-box approach with so-called "white-box" first-principles knowledge of objective function computation. Recent BO methods leverage such internal information to dramatically improve performance. For example, when optimizing a manufacturing line's throughput with simulation, we observe the number of parts waiting at each workstation, in addition to the overall throughput. However, internal information about objective function computation is often available. Classical BO methods assume that the objective function is a black box.

I haven't really noticed any difference while playing, other than the arm being in a different spot.Bayesian optimization (BO) is a framework for global optimization of expensive-to-evaluate objective functions. The tremolo unit is super close to the bridge, much closer than any other Jaguar I've played. The seller said they probably have the stock pickup sitting in a drawer somewhere but they couldn't find it. Honestly I wish it still had the stock Atomic Humbucker, as I'm a huge fan of those. The humbucker seems like it might be a bit too 80s metal for me, but I'll see how I go with it.
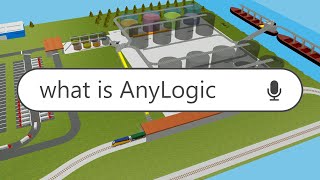
Further proof that Fender Mexico make excellent guitars.Ĭomes already upgraded with a Mastery bridge and a Wilde/Bill Lawrence L500-L humbucker. Right off the bat it feels much nicer to play than my MIJ Jag, and even sounds better acoustically.

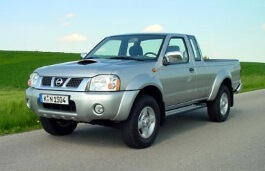
So I bought this one and I'll be selling the MIJ Jag. And here in front of me was a Jag in a beautiful finish with a humbucker. I don't use it much because I'd prefer it had humbuckers (which I'd need to route it for) and I don't really like the finish. My current Jag is a pretty standard MIJ reissue, stock Jag pickups and 3-tone-sunburst finish. It popped up on a local Facebook group and I thought "yeah that's cool, but I already have a Jag."īut then the trouble came when I thought about it more.
